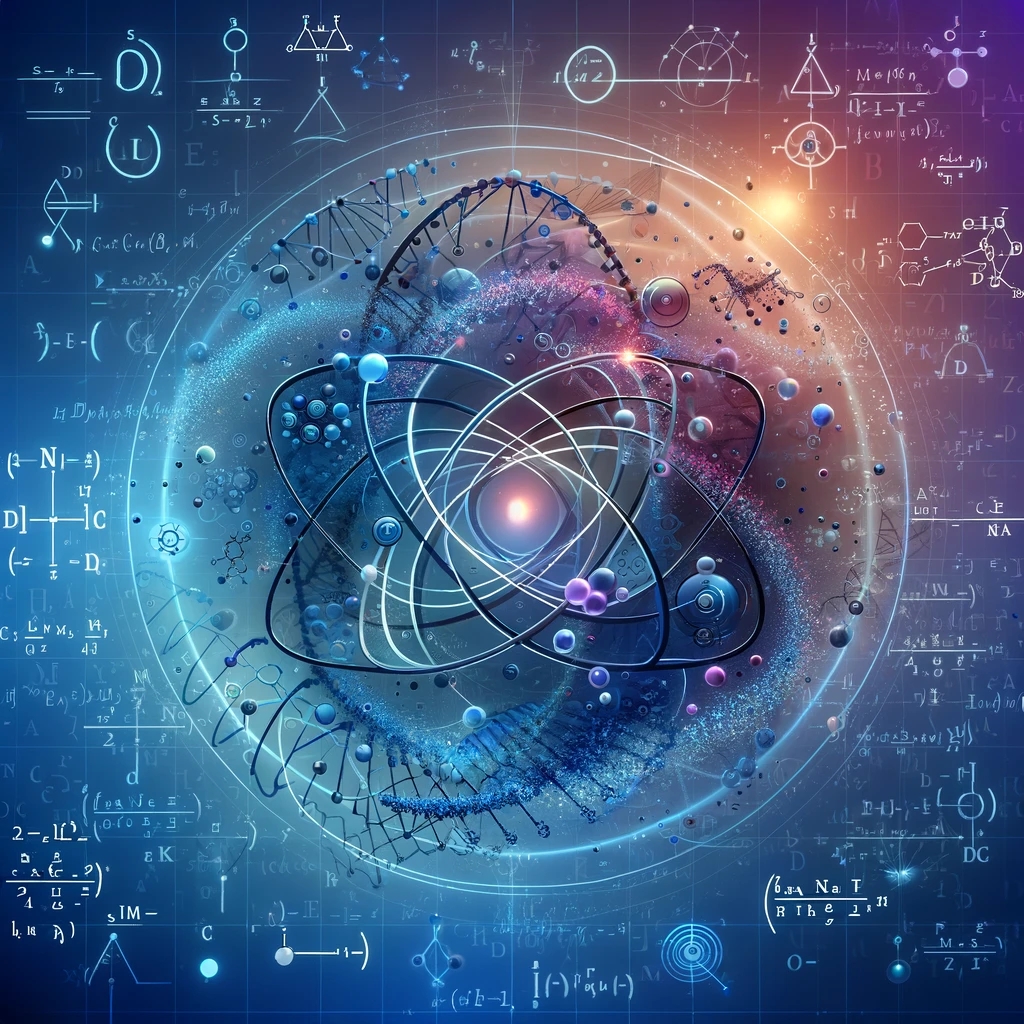
By: Kyle Alander
Table of Contents
Introduction
Understanding Ontological Commitments
Defining Proposition, Theory, Evidence and Its Universality
The Meaning of Science and Scientific Theories
Bayesian Epistemology as the Logic of Science
Scientific Theorizing as a Special Case of Bayesian Reasoning
Addressing Potential Objections
Introduction
In the quest to understand the world and our place within it, we often grapple with the question of what truly exists. These beliefs about what exists: our ontological commitments. Our Ontological commitments are the beliefs we hold about what entities and structures exist in the world, and determining these commitments is a fundamental aspect of forming a coherent worldview.. Determining these commitments is not a trivial task; it requires a rigorous, evidence-based approach to ensure that our beliefs are well-founded.
Within the philosophy of science and epistemology, various methods have been proposed for guiding our ontological commitments. One such method is to restrict these commitments to what our best scientific theories say. This is a sort of naturalistic epistemological view of belief formation where we restrict ourselves to empirical evidence and scientific theories as the basis for determining what exists. For the purposes of this, I will refer to this view as Scientific Ontologism. However, I will argue that another method of belief formation is superior: Bayesian epistemology. This method offers a more comprehensive and flexible framework for evaluating and updating our beliefs based on evidence. For these purposes, I shall refer to this alternative view as Bayesian Ontologism
In this blog, my thesis will be that our ontological commitments should be guided by a bayesian framework. I will demonstrate that the proposed method not only encompasses other views as special cases but also provides a unified and robust approach to determining what we should believe exists. By adopting this integrated framework, we can ensure that our ontological commitments are both epistemically sound and empirically grounded, it allows us to make more informed and rational decisions about our beliefs about what we think exist (our ontological commitments)
Understanding Ontological Commitments
Ontological commitments refer to the beliefs we hold about what entities and structures exist in the world. They form the foundation of our worldview, shaping our understanding of reality and influencing our decisions and actions. In other words, when we believe something exists we hold it as an ontological commitment Everyone’s worldview has one or more ontological commitments. And a coherent worldview depends on consistent and justifiable beliefs about what exists.
Now determining what our ontological commitments should be is not easy. Different philosophical perspectives offer competing views on what exists. For instance, materialism asserts that only physical entities exist, while dualism posits both physical and non-physical entities. These disagreements complicate the process of forming ontological commitments and the evidence for the existence of various entities and structures can be complex, indirect, and sometimes conflicting.
There are various methods for guiding our ontological commitments. Here though, I will focus on two primary approaches:
- Scientific Ontologism: This thesis states that we should restrict our ontological commitments to what our best scientific theories say. It relies on empirical evidence and the robustness of scientific theories to determine what exists.
- Bayesian Ontologism: This thesis states that our ontological commitments should be guided by what propositions we find to be most plausible based on the Bayesian method. It provides a comprehensive and flexible framework that can encompass scientific theories as well as other sources of evidence.
Now both methods make use of these criteria
Criteria for Ontological Commitments:
- Empirical Adequacy: Does the theory accurately describe and predict phenomena?
- Explanatory Power: Does the theory effectively explain the phenomena it addresses?
- Simplicity: Does the theory avoid unnecessary assumptions and entities?
- Coherence: Is the theory internally consistent and does it cohere with other well-supported theories?
I will argue that Bayesian Ontologism is Superior to Scientific Ontologism
Defining Proposition, Theory, Evidence and Its Universality
Defining Proposition
A proposition is a statement or assertion that expresses a judgment or opinion. It is a declarative sentence that can be either true or false. Propositions are the fundamental building blocks of theories, serving as the units of meaning that convey specific claims about the world.
Characteristics of Propositions:
- Truth-Value: Every proposition has a truth-value, meaning it can be evaluated as true or false.
- Declarative Nature: Propositions are declarative sentences that make specific claims.
- Components of Theories: Theories are composed of sets of propositions that collectively explain a particular phenomenon.
Examples Across Disciplines:
- History: Historical information can be expressed as propositions because propositions are statements about the past. For example, “The Roman Empire fell in 476 AD” is a historical proposition.
- Literature: “Hamlet is a tragic hero” is a literary proposition.
- Crime-Solving: “The suspect was at the scene of the crime” is a forensic proposition.
- Medical Diagnosis: “The patient has diabetes” is a medical proposition.
Theory Definition
A theory is a narrative (set of propositions) that attempts to tell a coherent and compelling story about the relevant data in such a way as to portray its probable truth. Theories are crucial tools for making sense of the world around us by providing structured explanations for observed phenomena. The concept of a theory is not confined to scientific inquiry; rather, it is a universal aspect of rational thought and analysis across various domains.
Applications Across Disciplines:
1. History: Theories in history aim to explain past events and trends based on available evidence, constructing a coherent narrative of the past.
2. Literature: Literary theories interpret texts to uncover deeper meanings and thematic structures, offering insights into the human experience and cultural context.
3. Crime-Solving: Theories in crime-solving reconstruct the events of a crime based on evidence, identifying perpetrators and understanding motives.
4. Medical Diagnosis: Medical theories diagnose illnesses by correlating symptoms and medical data with known conditions, creating a narrative of the patient’s health.
Universality of Theorizing:
Theorizing is common to all rational inquiry and is not exclusive to science. It reflects a rational creature’s attempt to make cognitive contact with the world. Regardless of the field, the goal of theorizing is to provide the most coherent and plausible explanation for the available evidence.
Defining Evidence
In the context of this discussion, we will adopt the positive relevance view of evidence. According to this view, evidence (e) is relevant for a hypothesis (h) if and only if the probability of h given e (p(h|e)) is greater than the probability of h without e (p(h)). In other words, e is evidence for h if and only if e raises the probability of h above what it was when e was not taken into account.
Positive Relevance Thesis:
– Definition: (e) is evidence for (h) if and only if p(h|e) > p(h) , meaning that (e) raises the probability of (h) above what it was without (e).
– Implication: This view emphasizes the role of evidence in increasing the likelihood of a hypothesis, making it a crucial component in the process of belief formation and theory evaluation.
Evidence Across Disciplines:
– History: Evidence includes historical documents, artifacts, and eyewitness accounts that support historical theories.
– Literature: Evidence consists of textual elements, themes, and patterns that support literary interpretations.
– Crime-Solving: Evidence includes forensic data, witness testimony, and physical clues that support criminal theories.
– Medical Diagnosis: Evidence involves clinical symptoms, test results, and patient history that support medical diagnoses.
Universality of Evidence Evaluation:
Evaluating evidence based on the positive relevance thesis is a universal aspect of rational inquiry. Whether in science, history, literature, crime-solving, or medical diagnosis, the goal is to determine how new evidence impacts the probability of a given hypothesis. This process ensures that our beliefs are continually refined and updated in light of new information.
By defining theory and evidence in this way, we establish a framework that is applicable across various fields of inquiry. This universality underscores the importance of a coherent and evidence-based approach to forming and updating our ontological commitments, which will be further explored in the context of Bayesian Ontologism.
The Meaning of Science and Scientific Theories
Etymology of Science
To understand the meaning of science, it’s helpful to start with its etymology:
- Origin: The word “science” comes from Old French, meaning knowledge, learning, application, and a corpus of human knowledge.
- Latin Root: Derived from the Latin word “scientia,” which meant knowledge, a knowing, expertness, or experience.
This historical perspective highlights that science fundamentally represents to collection of knowledge. While the meaning has changed over time and philosophers of science have no consensus on this question, generally its held that a scientific theory is a specific type of theory focused on empirical knowledge. It is a well-substantiated explanation of some aspect of the natural world that is based on a body of evidence and has stood up to repeated testing and scrutiny.
Using the previously defined concept of a theory as a narrative (set of propositions) that attempts to tell a coherent and compelling story about the relevant data in such a way as to portray its probable truth, we can derive an objective definition of a “scientific theory.”
Scientific Theory: a coherent set of propositions that aims to explain and predict natural phenomena based on empirical evidence.
We do not need to concern ourselves with debates about whether the entities posited in scientific theories exist; all that matters is that there is nothing exclusive about “scientific theories” that are logically different from other kinds of theories. While the “content” of a scientific theory will be different from the content of other kinds of theories (like the ones mentioned above), the actual meaning of a scientific theory as a set of propositions that try to tell a story is the same here as it is for any other theory within various domains of investigation.
Bayesian Epistemology as the Logic of Science
Bayesian epistemology provides a robust framework for evaluating and updating beliefs based on evidence, making it a cornerstone of rational inquiry. It is particularly valuable in the context of science, where hypotheses must be rigorously tested and revised in light of new data. Central to Bayesian epistemology is the concept of probabilistic degrees of support, which are mind-independent support relations connecting propositions.
Probabilistic Degrees of Support
Definition: Probabilistic degrees of support represent objective relationships between propositions, offering a structured way to determine how one proposition (such as evidence) probabilistically supports another (such as a hypothesis). These support relations are quantified as epistemic probabilities, which essentially measure the degree of support between propositions.
Atemporal Nature: Crucially, these relationships are atemporal. The value of a conditional probability, like P(A|B), is not influenced by the timing of discovering or understanding A and B. This means that the support one proposition offers another is independent of when they are discovered or understood.
Application in Hypothesis Evaluation:
When a hypothesis makes a prediction, this can be understood as a probabilistic degree of support of the hypothesis by the evidence. For instance, if a hypothesis predicts a particular outcome, and this outcome is observed, the observed evidence increases the probability of the hypothesis being true.
Theoretical Posits and Retroductive Inference
Theoretical Posits: In the advancement of scientific theorizing, theoretical posits are often essential. To explain observations (i.e., data), scientists may posit entities or mechanisms that are unobservable (i.e., theoretical). These posits represent propositions that can have evidential relationships, despite being unobservable.
Retroductive Inference: The inference process that underlines this notion is termed “retroductive inference,” initially formulated by Charles Sanders Peirce. Unlike standard deduction and induction, retroduction involves reverse-engineering the cause of an observed effect by forming hypotheses about the cause. This process often involves new ideas and may require new language as one moves from known effects (observables) to unknown causes (unobservables).
Importance in Science: Philosophers such as Ernan McMullin have argued that retroductive inference is fundamental to scientific inquiry. According to McMullin, retroduction is crucial because it allows scientists to generate new hypotheses and explanations for observed phenomena, facilitating the advancement of knowledge.
The Role of Retroductive Inference in Scientific Theories:
When we define a scientific theory as a coherent set of propositions that aims to explain and predict natural phenomena based on empirical evidence, we underscore the dynamic interplay between theoretical posits and empirical data. This process of hypothesizing about unobservable causes to account for observed effects is fundamental to scientific inquiry.
Theoretical Posits as Core Propositions of a Scientific Theory
Theoretical posits are central to scientific theories. These posits are propositions that form the core content of a theory, suggesting the existence of entities, structures, or mechanisms that are not directly observable. For example, in the theory of electromagnetism, theoretical posits include the existence of electric and magnetic fields. These posits provide a framework for understanding and explaining a wide range of phenomena, from the behavior of charged particles to the propagation of electromagnetic waves.
Predictions and Empirical Evidence:
A robust scientific theory not only includes theoretical posits but also makes specific predictions about observable phenomena. These predictions are propositions about what we should expect to find if the theory is accurate. For instance, the theory of electromagnetism predicts that a changing magnetic field will induce an electric current in a conductor. When these predicted phenomena are observed, the empirical evidence supports the theoretical posits of the theory.
The relationship between theoretical posits and empirical evidence is captured through probabilistic degrees of support. When a prediction derived from the theoretical posits is confirmed by observation, the probability of the theory being true increases. This is because the observed evidence (e) raises the probability of the hypothesis (h), formalized as P(h|e) > P(h) . Thus, the evidence supports the theory by confirming the predictions made by its core propositions.
Integration with Bayesian Epistemology
Bayesian epistemology offers a structured framework for evaluating the probabilistic support between propositions, making it particularly well-suited to the process of retroductive inference. By quantifying the degree of support that empirical evidence provides to theoretical posits, Bayesian reasoning ensures that our scientific theories are rigorously tested and updated.
In this framework, a scientific theory is not static; it evolves as new evidence is gathered. The theoretical posits are continually tested through their predictions, and as empirical support accumulates, the theory becomes more robust. Conversely, if predictions fail, the theory must be revised or replaced. This iterative process, guided by Bayesian principles, ensures that scientific theories remain dynamically aligned with empirical reality.
By taking retroductive inference seriously, we recognize that the theoretical posits within a scientific theory are propositions that aim to explain natural phenomena. These posits generate predictions about other propositions, which, if confirmed by empirical evidence, support the content of the theory. This interplay between theoretical hypotheses and empirical testing is at the heart of scientific inquiry, driving the advancement of knowledge and ensuring that our ontological commitments are both coherent and evidence-based. Through Bayesian epistemology, we can systematically evaluate and update these commitments, maintaining a rigorous and dynamic approach to understanding the natural world.
Bayesian Epistemology: Bayesian epistemology integrates these concepts into a comprehensive framework for scientific reasoning. By evaluating hypotheses based on their probabilistic support and continuously updating beliefs as new evidence emerges, Bayesian reasoning ensures that our scientific theories remain robust and empirically grounded.
Logical Foundation of Science:
Bayesian epistemology provides the logical foundation for scientific theorizing. It explains how we should update our beliefs in light of new evidence, ensuring that our ontological commitments are both epistemically sound and empirically justified. This approach aligns with the scientific method’s emphasis on evidence and rigorous testing, making it an essential tool for rational inquiry.
By understanding Bayesian epistemology as the logic of science, we can appreciate its role in guiding our ontological commitments and ensuring that our beliefs are grounded in a coherent and evidence-based framework. This integration of probabilistic reasoning, theoretical posits, and retroductive inference forms the backbone of scientific inquiry, facilitating the continual advancement of knowledge.
Scientific Theorizing as a Special Case of Bayesian Reasoning
Bayesian reasoning provides a comprehensive framework for evaluating and updating beliefs based on evidence. Within this framework, scientific theorizing can be seen as a specific application of Bayesian principles. This view highlights the deep connection between the methodologies of science and the broader logic of Bayesian epistemology.
Bayesian epistemology is a method of reasoning that uses probabilities to represent the degree of belief in a proposition given certain evidence. It is grounded in Bayes’ theorem, which provides a mathematical formula for updating these probabilities as new evidence becomes available. The general form of Bayes’ theorem is:
P(H|E) = [P(E|H) x P(H)/P(E)]
where:
– P(H|E) is the posterior probability of the hypothesis (H) given the evidence (E).
– P(E|H) is the likelihood of the evidence given the hypothesis.
– P(H) is the prior probability of the hypothesis before considering the evidence.
– P(E) is the marginal probability of the evidence.
This framework allows for the systematic and continuous updating of beliefs, ensuring that our ontological commitments are both dynamic and responsive to new information.
Scientific Theories as a Special Case:
Scientific theories, when viewed through the lens of Bayesian reasoning, are special cases where the propositions and evidence are structured and formalized within the scientific method. Scientific theorizing involves several steps that align closely with Bayesian principles:
1. Hypothesis Formation: Scientists begin by formulating hypotheses or theoretical posits based on initial observations. These hypotheses represent propositions about unobservable entities or mechanisms that explain the observed phenomena.
2. Prediction and Testing: Hypotheses generate predictions about observable outcomes. These predictions are tested through systematic observation and experimentation, yielding empirical data.
3. Evidence Evaluation: The empirical data is evaluated to determine how well it supports the hypotheses. This is where Bayesian reasoning comes into play, as the data (evidence) is used to update the probability of the hypotheses being true.
4. Posterior Update: Using Bayes’ theorem, scientists update their beliefs about the hypotheses. If the evidence strongly supports the hypotheses, the posterior probability increases. Conversely, if the evidence contradicts the hypotheses, the probability decreases.
Scientific theorizing as a special case of Bayesian reasoning can be understood through the logical reduction of scientific processes to Bayesian updates. The process of hypothesis testing in science mirrors the Bayesian process of updating beliefs based on evidence:
– Prior Probability: In science, the prior probability reflects the initial plausibility of a hypothesis based on existing knowledge and theory.
– Likelihood: The likelihood corresponds to the predicted outcomes of the hypothesis. It measures how probable the evidence is, assuming the hypothesis is true.
– Posterior Probability: The posterior probability represents the revised plausibility of the hypothesis after considering the new evidence.
By applying Bayesian principles, scientific theories are continuously refined and improved, ensuring that they remain aligned with empirical reality. This iterative process of prediction, testing, and updating is fundamental to both scientific inquiry and Bayesian reasoning.
Benefits of Integration
Viewing scientific theorizing as a special case of Bayesian reasoning offers several benefits:
1. Unified Framework: It provides a unified framework for understanding how scientific knowledge is developed and validated. This integration emphasizes the continuity between everyday reasoning and scientific inquiry.
2. Epistemic Rigor: Bayesian reasoning enhances the epistemic rigor of scientific theories by providing a clear and systematic method for evaluating and updating beliefs based on evidence.
3. Dynamic Adaptability: Both Bayesian reasoning and scientific theorizing are inherently dynamic, allowing for continuous adaptation and improvement as new evidence emerges.
4. Parsimony and Ontological Economy: Bayesian principles favor simpler, more plausible hypotheses, which aligns with the scientific preference for parsimonious explanations.
Logical Reduction
Given everything discussed so far, now comes the critical part of this blog: showing that scientific ontologism just reduces to Bayesian ontologism.
Scientific Ontologism
Scientific ontologism is the view that our ontological commitments should be restricted to what our best scientific theories say. This approach emphasizes the importance of empirical evidence and the robustness of scientific theories in determining what exists. According to scientific ontologism, the entities and structures posited by well-supported scientific theories are the only ones we should commit to ontologically.
Bayesian Ontologism
Bayesian ontologism, on the other hand, is the thesis that our ontological commitments should be guided by what propositions we find to be most plausible based on the Bayesian method. This method involves evaluating and updating beliefs based on the probabilistic support provided by evidence. Bayesian ontologism is a broader framework that can encompass scientific theories as well as other sources of evidence.
Logical Reduction of Scientific Ontologism to Bayesian Ontologism
To show that scientific ontologism logically reduces to Bayesian ontologism, we can use the definition of a “scientific theory” as a coherent set of propositions that aims to explain and predict natural phenomena based on empirical evidence to show the logical deduction:
1. Empirical Evidence and Bayesian Updating:
– Scientific Theory Definition: A scientific theory is a set of propositions based on empirical evidence.
– Bayesian Context: Bayesian ontologism values empirical evidence within a broader probabilistic framework, where evidence is used to update the probabilities of hypotheses.
– Reduction: The process of updating scientific theories based on empirical evidence aligns with Bayesian updating. Both rely on empirical data to revise the probabilities of propositions, ensuring our beliefs are aligned with the best available evidence.
2. Robustness of Scientific Theories:
– Scientific Theory Definition: Scientific theories are well-substantiated explanations or narratives of natural phenomena that withstand rigorous testing.
– Bayesian Context: In Bayesian terms, a theory repeatedly confirmed by evidence has a high posterior probability.
– Reduction: The robustness of scientific theories is captured by the high posterior probabilities assigned to them in Bayesian reasoning, justifying ontological commitments to their posited entities and structures.
3. Predictive Power and Likelihood:
– Scientific Theory Definition: Scientific theories aim to predict natural phenomena.
– Bayesian Context: Bayesian reasoning evaluates the likelihood of evidence given a hypothesis. A theory with strong predictive power has a high likelihood (P(E|H).
– Reduction: When a scientific theory’s predictions are confirmed, the observed evidence increases the posterior probability of the theory, aligning predictive power with Bayesian likelihood evaluation.
4. Falsifiability and Probabilistic Support:
– Scientific Theory Definition: Scientific theories must be testable and falsifiable.
– Bayesian Context: Bayesian reasoning involves updating beliefs based on new evidence, similar to the concept of falsifiability.
– Reduction: The ability to test and potentially disprove scientific theories is mirrored in Bayesian reasoning’s requirement to update probabilities based on new evidence, maintaining the epistemic soundness of hypotheses.
5. Ontological Economy and Parsimony:
– Scientific Theory Definition: Scientific theories prefer simpler, more parsimonious explanations.
– Bayesian Context: Bayesian reasoning incorporates parsimony through prior probabilities, assigning higher priors to simpler hypotheses.
– Reduction: The preference for parsimony in scientific theories aligns with Bayesian principles, where simpler hypotheses are favored unless strong evidence supports more complex ones.
By integrating these principles, we see that scientific ontologism’s reliance on empirical evidence, robustness, predictive power, falsifiability, and parsimony are all encapsulated within the Bayesian framework. Scientific ontologism thus logically reduces to Bayesian ontologism, as the latter provides a comprehensive method for evaluating and updating all ontological commitments based on probabilistic support.
We can further support this by understanding reproductive inference:
Logical Reduction of Scientific Ontologism to Bayesian Ontologism through Retroductive Inference
Retroductive inference, or abduction, is essential in scientific theorizing. It involves reasoning from observed effects to hypothesized causes, forming the basis of many scientific theories. By examining retroductive inference through the lens of Bayesian reasoning, we can show that scientific ontologism logically reduces to Bayesian ontologism.
Retroductive inference begins with observing certain effects and positing unobservable causes to explain these effects. Bayesian reasoning similarly involves forming hypotheses based on observed evidence. When we observe data, we hypothesize potential causes and use Bayesian methods to evaluate and update the probabilities of these hypotheses. This process aligns retroductive inference with Bayesian inference, demonstrating that the retroductive process in scientific theorizing is inherently Bayesian.
Scientific theories are evaluated by their ability to make accurate predictions about observable phenomena. In Bayesian terms, this involves assessing the likelihood of the evidence given the hypothesis P(E|H). Confirmed predictions increase the posterior probability P(H|E).
Retroductive inference posits theoretical entities to explain observed phenomena, which are tested through their predictive power. Bayesian reasoning formalizes this by updating the probabilities of theoretical posits based on evidence. Thus, the process of validating theoretical posits in scientific ontologism is encapsulated within the Bayesian framework.
Falsifiability and Bayesian Updating:
Falsifiability is a key criterion for scientific theories, ensuring they can be tested and potentially disproven. Retroductive inference involves forming testable hypotheses, which are revised or rejected if contradicted by evidence. This concept mirrors Bayesian reasoning, where beliefs are continuously updated based on new evidence.
In scientific ontologism, falsifiability ensures that only empirically supported entities are included in our ontological commitments. Bayesian updating serves the same function, aligning beliefs with the best available evidence. Therefore, falsifiability in scientific theorizing reduces to Bayesian updating.
Ontological Economy and Bayesian Parsimony:
Scientific ontologism values parsimony, preferring simpler theories with fewer ontological commitments. Retroductive inference involves positing the minimal necessary theoretical entities (minimal theoretical content of a theory) to explain observed phenomena. Bayesian reasoning thus inherently incorporates parsimony through prior probabilities, assigning higher priors to simpler hypotheses that make less theoretical atomic propositions. This alignment shows that the parsimony valued in scientific ontologism is a direct application of Bayesian principles.
By examining retroductive inference through the lens of Bayesian reasoning, we see that the principles of scientific ontologism—empirical evidence, robustness, predictive power, falsifiability, and parsimony—are all encapsulated within the Bayesian framework. The process of forming, testing, and updating theoretical posits through retroductive inference aligns seamlessly with Bayesian updating, demonstrating that scientific ontologism logically reduces to Bayesian ontologism.
Answering Potential Objections
Objection 1: Bayesianism and Content
Objection: Bayesianism is a method for updating beliefs based on evidence, but it doesn’t inherently determine what the content of those beliefs should be. Scientific theories provide a specific and empirically grounded set of propositions that we can commit to, while Bayesianism alone doesn’t specify what these propositions are.
Response:
While Bayesianism does focus on the method of updating beliefs, it still inherently involves evaluating the plausibility of propositions based on evidence. The content of the propositions is part of the Bayesian framework because the propositions themselves contain the content which are evaluated and updated. The best scientific theories are already examples of propositions that have been evaluated and updated using Bayesian reasoning. By applying Bayesianism universally, we are not rejecting scientific theories but integrating them into a broader framework that can encompass other well-supported propositions.
Objection 2: Specificity of Scientific Theories
Objection: Scientific theories are specific and have a track record of success in explaining and predicting phenomena. Bayesianism is too general and abstract to serve as the sole basis for ontological commitments.
Response:
Bayesian reasoning provides the foundational logic for evaluating and updating scientific theories. It is not abstract in a way that undermines specificity but rather underpins the very success of scientific theories by ensuring they are grounded in evidence and updated accurately. Bayesianism can be applied specifically to evaluate scientific theories, ensuring that our ontological commitments remain robust and well-supported. It enhances rather than diminishes the specificity and success of scientific theories.
Objection 3: Potential for Question-Begging
Objection: Relying solely on Bayesianism to filter ontological commitments might be seen as question-begging if it implicitly favors certain types of propositions (e.g., scientific ones) over others without proper justification.
Response:
Bayesianism does not inherently favor any specific type of proposition. It evaluates all propositions based on their evidence and plausibility. This universality ensures that no specific ontology is assumed a priori. This is in contrast to the scientific ontologist which does assume a specific ontology a priori. However scientific propositions often fare well under Bayesian evaluation because of their empirical success and explanatory power. This is not question-begging but a reflection of their robust evidential support. Bayesianism simply provides a fair and rigorous method for this evaluation.
Objection 4: Ontological Economy
Objection: Ontological economy suggests we should prefer theories that make the fewest assumptions. Scientific theories are more parsimonious as they avoid positing supernatural entities, whereas Bayesianism might allow for a broader range of ontological commitments.
Response:
Bayesian reasoning inherently incorporates the principle of parsimony by favoring simpler, more plausible propositions. It doesn’t require additional assumptions unless they are strongly supported by evidence. Bayesianism allows for a comprehensive evaluation of all propositions, ensuring that any ontological commitment, whether scientific, historical or otherwise, is justified based on its evidential support and simplicity.
By addressing these objections, we demonstrate that Bayesian epistemology provides a robust and universal framework for making ontological commitments. It not only supports the success of scientific theories but also ensures that our beliefs are grounded in the most plausible and well-supported propositions, regardless of their content. This approach aligns ontological commitments with the principles of evidence, simplicity, and coherence, enhancing the reliability and adaptability of our understanding of the world.
Conclusion: A Bayesian Approach to Ontological Commitments
Given everything discussed in this blog, we now have a universal way to evaluate our ontological commitments through the lens of Bayesian epistemology. By applying Bayesian reasoning, we can ensure that our beliefs are grounded in the most plausible and well-supported propositions, regardless of their content. This approach aligns our ontological commitments with the principles of evidence, simplicity, and coherence, enhancing the reliability and adaptability of our understanding of the world.
Bayesian epistemology can effectively ground the criteria for ontological commitments:
1. Empirical Adequacy:
– Bayesian reasoning emphasizes updating beliefs based on evidence. A theory’s empirical adequacy—its ability to describe and predict (or retrodict) phenomena accurately—directly influences its likelihood. As evidence accumulates in favor of a theory’s predictions, its posterior probability increases, indicating strong empirical support.
2. Explanatory Power:
– The explanatory power of a theory can be seen in how well it accounts for the available evidence compared to alternative theories. In Bayesian terms, this is reflected in the likelihood ratio P(E|H) / (P(E|~H), where (E) is the evidence and (H) is the hypothesis. A theory that provides a better explanation for the evidence will have a higher likelihood ratio, making it more probable after updating.
3. Simplicity (Ontological Economy):
Simplicity is incorporated into Bayesian reasoning through prior probabilities. Simpler theories, which make fewer assumptions and posit fewer entities, are often assigned higher prior probabilities because they adhere to the principle of parsimony (Occam’s Razor). When updating beliefs, a simpler theory with a reasonable fit to the evidence may be preferred over a more complex one with a marginally better fit due to its higher prior probability.
4. Coherence:
Coherence refers to a theory’s internal consistency and its consistency with other well-supported theories. In Bayesian terms, coherence can be considered when setting prior probabilities and evaluating the joint probability of multiple related hypotheses. A theory that is internally consistent and aligns well with established theories will have a higher joint probability, making it more likely to be accepted after Bayesian updating.
In conclusion, Bayesian epistemology provides a robust framework for evaluating and updating our ontological commitments. By grounding our commitments in empirical adequacy, explanatory power, simplicity, and coherence, we ensure that our beliefs are both plausible and well-supported. This comprehensive and rigorous methodological framework allows us to navigate the complexities of belief formation and maintain a coherent and adaptable understanding of the world.
By addressing potential objections and demonstrating how Bayesian reasoning integrates scientific theories and broader sources of evidence, we can see that Bayesian ontologism offers a unified and superior method for determining what we should believe exists contra Scientific Ontologism. This approach not only supports the success of scientific theories but also ensures that our ontological commitments remain flexible, dynamic, and responsive to new evidence, ultimately leading to a more reliable and coherent worldview.
_______________________________________
Footnotes:
- Scientific Ontologism and Bayesian Ontologism are terms that I coined
- Roush†, Sherrilyn (2004). Discussion note: Positive relevance defended. Philosophy of Science 71 (1):110-116.
- Nevin Climenhagas work has been the most updated model in understanding this view, see Nevin Climenhaga. Epistemic probabilities are degrees of support, not degrees of (rational) belief. Philosophy and Phenomenological Research. 2023;1-24.
- The temporal view assumes that the timing of discovering evidence is epistemically relevant. For instance, one version of this would say that forward-looking predictions generate stronger epistemic reasons than do backward-looking ones. This view is called “predictivism.” For an argument against it, see Swinburne (2001: 221–232, appendix on Predictivism).
- McMullin, Ernan (1992). The inference that makes science. Milwaukee: Marquette University Press.
- Note: The theory of parsimony I am assuming is that theories with less content or less independent atomic propositions in the theoretical content are simpler and more probable all things being equal
are you knowledgeable in the gospels history and historical criticism I wanted to ask you about something I read something by a Jesus mysticist bothered me on one Thessalonians 2: 13 to 16
LikeLike